Research roundup: Enhancing GNSS resilience
GNSS researchers presented hundreds of papers at the 2023 Institute of Navigation (ION) GNSS+ conference, which took place Sept. 11-15, 2023, in Denver, Colo., and virtually.
The following four papers focused on ways to combat GNSS jamming and spoofing. The papers are available here.
GPS World will attend this year’s ION conference in Baltimore, Maryland on Sept. 16-20.
Approximating Regional GNSS Interference Sources Using ADS-B Data
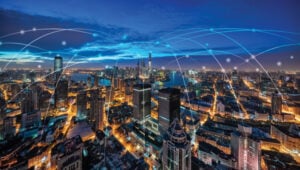
Photo: zhanghaitao / iStock / Getty Images Plus / Getty Images
The Automatic Dependent Surveillance-Broadcast (ADS-B) system, widely used for air traffic operations and management, also has potential applications in identifying, detecting and localizing (IDL) GNSS/RFI jamming sources in regions with high air traffic. With the rise in global GNSS interference reports, it is crucial to identify and eliminate jammers to ensure safe air travel operations.
The Navigational Integrity Category (NIC) value included in the ADS-B message is a key indicator for detecting potential jamming from ADS-B data. Although NIC is not the most effective metric for interference detection, it can still signal the presence of jamming and offer a means to localize the source in real time.
This research aims to approximate the area of GNSS/RFI interference by fitting a Euclidean Cone to ADS-B data that reports low NIC values. The problem is formulated as a convex optimization problem, derived from an alternative version of the maximum inscribed ellipsoid approach. By fitting an optimal cone to the data affected by interference, the cone’s apex indicates the estimated jamming location. The research team processed, decoded, interpolated and filtered ADS-B data to enhance localization accuracy.
The proposed convex formulation was tested on two reported interference events: one near Denver International Airport in January 2022, for 36 hours, and another near the Dallas-Fort Worth area in October 2022, over roughly eight hours. In Denver, four estimated jamming locations, calculated from four six-hour time windows, were grouped between downtown Denver and the airport. In Dallas, three estimated jamming locations, determined from three one-hour windows, showed a tighter grouping on the southern side of the Dallas/Fort Worth area, indicating spoofing was nearby.
Michael Dacus, Zixi Liu, Sherman Lo and Todd Walter, “Approximating Regional GNSS Interference Sources as a Convex Optimization Problem Using ADS-B Data.”
Hybrid Autoencoder for Interference Detection
Malfunctions or failures in GNSS services can result in significant personal, material, and financial damages. Early identification of anomalous behavior in GNSS signals can enable timely countermeasures. However, many interference monitoring or mitigation techniques are only feasible with high-end receivers and demand a certain level of expertise to be used effectively.
This paper presents a GNSS interference monitoring approach employing machine learning methodologies for users of any expertise level and with any type of GNSS receiver capable of outputting raw GNSS observations. The research team used simple signal-to-noise ratio (SNR) observations and different hybrid autoencoder models, including denoising or variational autoencoder combined with recurrent neural network (RNN) models, which are trained and tested on real jamming and spoofing events. The developed monitoring system is represented by a “traffic lights” system, indicating the severity or level of concern associated with each detected anomaly.
The results compare different RNN-based autoencoder implementations and have been tested on input data from high-end to low-end GNSS receivers. The analysis of the test set showed that there is a 95 percent probability of catching anomalies. Additionally, similar results were achieved when applied to other geodetic receiver types such as u-blox or JAVAD GNSS receivers. However, smartphone data is subject to some limitations. Notably, missed anomalies are primarily attributed to the low transmitting power from the jamming and spoofing devices, which poses challenges for detection.
Karin Mascher, Stefan Laller and Philipp Berglez, “Hybrid Autoencoder for Interference Detection in Raw GNSS Observations.”
A Tool to Monitor, Analyze and Record Navigation Signals
Given the heavy reliance on GNSS for numerous critical applications, any disruption caused by intentional or unintentional RFI could pose significant threats to operations that depend on these systems, from transportation and logistics to emergency services and national security. Developing advanced countermeasures against RFI has become a priority to ensure the functionality and resilience of GNSS-dependent systems.
This paper presents an architecture for real-time detection and classification of RFI affecting multi-band GNSS signals based on a machine learning method. The study proposes an architecture combining an actual GNSS monitoring station for recording GNSS signals…