Precise positioning for autonomous vehicles
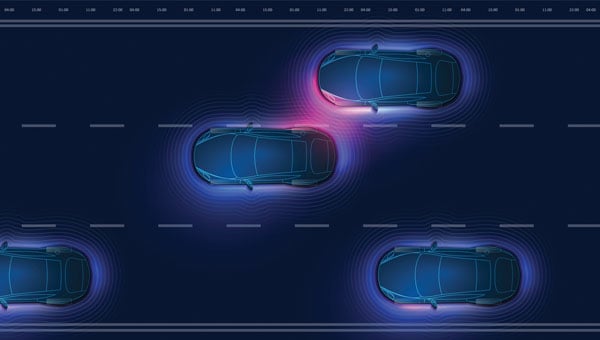
Photo: NatalyaBurova / iStock / Getty Images Plus / Getty Images
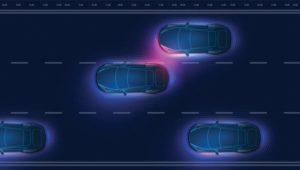
Photo: NatalyaBurova / iStock / Getty Images Plus / Getty Images
GNSS researchers presented hundreds of papers at the 2024 Institute of Navigation (ION) GNSS+ conference, which took place Sept. 16-20 in Baltimore. The following papers focus on high-accuracy positioning for autonomous vehicles in various environments. The papers are available here.
High-accuracy and resilient GNSS receiver for autonomous vehicles
The G3STAR GNSS receiver, a key component of the GAMMS Horizon 2020 project, is designed to improve high-definition navigation map production for autonomous vehicles. This Galileo-based receiver leverages the constellation’s Open Service features, including the High Accuracy Service (HAS) and Navigation Message Authentication (OSNMA). The research team shared that G3STAR’s ability to obtain and decode HAS messages from Galileo E6-B signals, as well as to process OSNMA bits from live Galileo E1-B I/NAV messages, demonstrates its advanced capabilities in providing secure and precise navigation data.
Preliminary tests highlight G3STAR’s proficiency in utilizing Galileo’s new services. However, the research team shared that further evaluation is necessary to fully assess its impact within the GAMMS project. Plans include validating the HAS data’s effect on navigation accuracy, conducting field tests to evaluate OSNMA availability in various environments and assessing the influence of the Chip Scale Atomic Clock on receiver performance. Additionally, comparing the G3STAR’s performance to commercial off-the-shelf receivers will be crucial in determining its overall contribution to the GAMMS navigation system and HD map generation. These evaluations will be carried out during upcoming test campaigns, providing valuable insights into G3STAR’s potential to advance autonomous vehicle navigation.
Filipe Carvalho, Ricardo Prata, Bruno Cardeira, Carlota Cardoso, Rui Nunes and António Fernández; “High-Accuracy and Resilient GNSS Receiver for an Autonomous Vehicle.”
GNSS/INS positioning software library
The autonomous vehicle industry has seen significant interest and investment throughout the past 15 years, with numerous practical applications emerging in the market. However, the technology for functionally safe GNSS/INS localization in autonomous vehicles is still not fully established. This gap is particularly crucial in safety-critical applications, where positioning algorithms must be robust against potential faults, especially in challenging environments. This paper highlights Hexagon’s Safety-Critical Positioning Solution, which addresses this need by providing both precision and safety for autonomous land vehicles.
The Positioning System is a safety-first software library that integrates GNSS signals, state space corrections from the TerraStar-X Enterprise service, inertial measurement units (IMUs) and additional vehicle sensors. This system employs an extension of Receiver Autonomous Integrity Monitoring techniques, originally developed for the aviation industry. It computes multiple navigation solutions using a solution separation technique, including an “all-in-view” solution and several subset solutions that exclude various fault hypotheses. These solutions are used to calculate Protection Levels (PLs), which provide an estimated upper bound on positioning errors, accounting for systematic biases and measurement faults. The PLs can be compared against alert limits to determine whether the navigation solution is sufficiently accurate for autonomous decision-making.
Eduardo Infante, Rudi Gaum and Laura Norman; “Demonstration of a Functionally Safe GNSS/INS Positioning Software Library for Autonomous Land Vehicles.”
Unmanned ground vehicles in off-road environments
This paper explores the emerging potential of radar for localization in GNSS-denied scenarios, particularly in challenging off-road environments where lidar-based systems struggle. The research focuses on two distinct settings: a dense forest and an underground mine. To address the localization challenges in these environments, the team developed a pipeline that combines an adaptive extended Kalman filter (EKF) for unstructured forested regions with a factor graph approach that fuses EKF estimates and point-to-plane radar iterative closest point (ICP) measurements for structured underground environments. The results demonstrate significant improvements in localization accuracy compared to existing methods, with the adaptive EKF proving particularly effective in forested areas.
The study provides valuable insights into the integration of radar and IMU data for vehicle localization in GPS-denied scenarios. While the adaptive EKF outperformed conventional EKF in structured outdoor settings, the standard EKF showed better performance in the highly dynamic conditions of the underground mine. The factor…